There’s a growing body of evidence that diversity & inclusion (D&I) practices in the workplace can pay dividends. Organizations that are intentional about their diversity profile:
- Gain access to a wider range of skill sets than organizations that confine their talent searches to “just like me” pools.
- Fulfill an important social mandate to eliminate discrimination.
- Reflect customer demographics authentically, leading to better user experiences.
- Are highly desirable places to work because they have a positive and inclusive work culture.
- Lead their communities and competitors by example.
- Achieve a better bottom line.
Research speaks volumes – diversity is a good thing. But how do you get there? The inequities we see in many organizations are multifactorial and not always the result of poor HR practices. In fact, many organizations have done their best to be “blind” to identity markers. But even when individuals do their best to eliminate bias, systemic biases may undermine these good intentions.
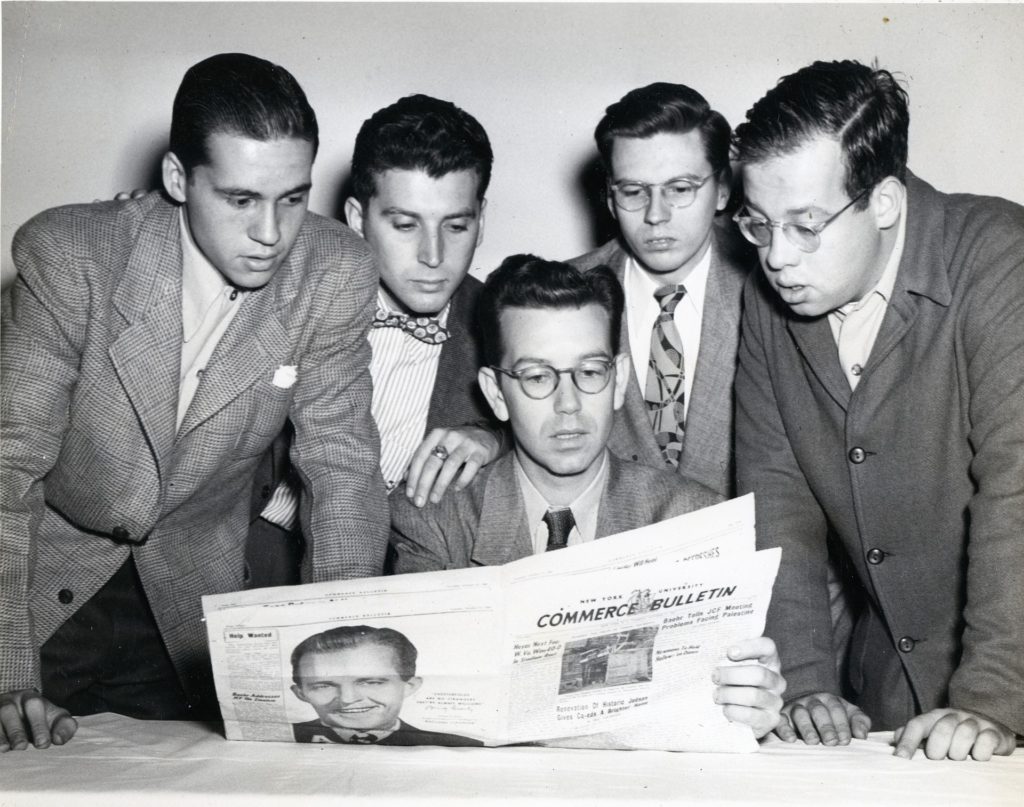
For example, an organization may consider its work culture positive because people are like-minded, get along with each other and play sports or watch games together – but fail to notice that what’s actually developing is a “bro culture” that may be intimidating to women. Leadership may tell themselves that this culture arose organically, when in reality the organization is putting out subtle cues about who fits in and who doesn’t.
We’re only human. We deal with individuals, not demographics, so we may not see these types of dynamics even when they’re right in front of us. Especially when an organization is too small for its makeup to be statistically significant, we may not see patterns emerging. And when we do look, we get uncomfortable, because these are people, not numbers or statistics, and we’d rather tell ourselves the story that we’re oblivious to race, gender, orientation or other characteristics.
So how do we proactively encourage diversity and inclusion without making judgments based on identity markers?
Artificial intelligence (AI) is a tool that can be leveraged throughout the HR talent cycle:
- Attraction/recruitment
- Hiring
- Compensation
- Development, reskilling, upskilling
- Evaluation
- Termination
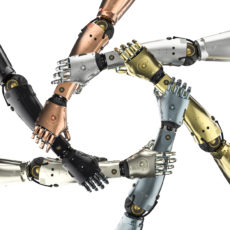
Each of these facets of employment is subject to bias, whether implicit or explicit.
Making opportunities more inclusive
Online job search mechanisms rely on keywords; job seekers enter what they assume to be relevant words and receive a list of possible roles. This can be limiting – the words entered may be too broad (e.g., “communication” is a function of almost all jobs), and paradoxically too narrow (e.g., by specifying “communication,” the job seeker hits on roles such as web developer but misses a host of other roles involving communication). What if an AI could engage in a chat with the job-seeker to determine his or her interests? A series of questions could help zero in on the job seeker’s interests, abilities, and potential – based on which, the AI could suggest specific roles. This creates inclusivity by addressing the information asymmetry that exists between insiders and outsiders of a given industry (i.e., people already in the industry know about the roles and what’s required to do them and can list relevant keywords, but industry outsiders are missing this valuable information).
Making job descriptions more inclusive
Studies show that job postings contain subtle cues that can attract certain candidates and deter others. For instance, a posting may use gender-coded words such as “ninja warrior” or “rockstar,” or emphasize a competitive culture where employees race to work hardest and stay the longest each day. This can deter parents – especially single parents, and often by extension, socioeconomically challenged applicants. Long lists of “must-haves” can also be a deterrent; studies show that when confronted with such lists, men will apply regardless of whether they have the full package, whereas women will avoid applying unless they feel they can satsify all requirements. Similarly, postings with a lot of jargon signal an “in-group”mentality, which may make their respective industries seem impenetrable. This can deter people who may lack experience but have potential from applying.
AI can play a role in parsing job postings for this type of coded language to ensure descriptions and requirements are gender-neutral and welcoming to all. It can also assess skill sets to determine which are job-critical and which are nice-to-have.
Helping companies look “blindly” at resumes
Research suggests employers have a tough time remaining impartial to signals and cues in job applications and resumes. Especially in high-tech fields, hiring managers may lean toward hiring younger applicants and weed out older applicants based on clues such as long date ranges in their CVs. They may unconsciously (or sometimes consciously) cull applicants whose names suggest particular ethnicities, or favour others based on stereotypical notions about the abilities of particular races or ethnic groups. One American study found that “whitened” resumes –scrubbed of clues as to race or ethnicity – resulted in over twice as many call-backs as resumes containing such markers. Even when companies advertised themselves as inclusive organizations, many applicants were reluctant to include details that might suggest their ethnicities.
AI has some potential for “blinding” – i.e., it can be used to mask off certain details such as names, ages and even postal codes. However, caution is warranted, as AI sorting can end up reinforcing bias rather than eliminating it (consider Amazon’s infamous algorithm based on “machine learning” that men have more experience in technical roles, and the resultant “sexist AI” that penalized resumes containing references to women).
Minimizing bias throughout an employee’s tenure
There are many opportunities along the HR cycle to develop, reward or promote an employee. Companies that are able to identify potential in existing employees and map out career paths are able to achieve cost savings over those that look outside the organization for talent. Skill development can enhance retention of employees by making them feel appreciated and included, which contributes to positive teams and culture.
The flipside is that sometimes organizations need to discipline or let an employee go. It’s imperative that these decisions be made fairly and without bias.
There may be some potential for AI to help map skill sets to opportunities as they arise within an organization. The role AI plays in eliminating hiring/firing bias remains to be seen, and can be expected to evolve as machine learning becomes more sophisticated.
Caveats
It should never be forgotten that AI is fuelled by data. AI “learns” by consuming large datasets of information, by which it establishes baselines and norms. This information comes from humans and is thus curated from the get-go. The saying “garbage in, garbage out” may very well apply here if the training datasets are poor or inaccurately representative.
When AI receives poorly curated training data the result can be a magnification of bias rather than a mitigation. This is especially true of historical data. For example, if a company has often hired Asian workers to do a particular task, the AI may embed the assumption that “Asians are best at this task.” It may be more helpful to develop aspirational algorithms rather than lean on historical data if the goal is to make changes to HR practices and be truly neutral.
Thus AI training (machine learning) is a delicate task and a great responsibility. It shouldn’t be undertaken without a coherent sense of what the goals are for a diversity strategy. It’s especially important to note that programmers typically aren’t trained in D&I, and they are human beings, so they carry the risk of introducing their own biases to AI. If you’re unsure about how to bring in the right expertise, consider meeting with a diversity & inclusion consultant.
Lastly, transparency is crucial. Any AI vendor you consider needs to be able to tell you how its machine learning and algorithms work. Using AI to support more diverse and inclusive hiring practice doesn’t mean taking a hands-off approach. It means leveraging technology so you can create a better workplace.
Sources: Harvard Business Review and The Washington Times
Diversation Question
How do you feel about AI in the workplace? Do you think it will make HR practices more diverse and inclusive? Drop us a line in the comments.